Database Walkthrough¶
[1]:
import os
import numpy as np
import pandas as pd
import seaborn; seaborn.set_theme()
import plotly.io; plotly.io.templates.default = "seaborn"
import emat
import yaml
from emat.util.show_dir import show_dir
from emat.analysis import display_experiments
emat.versions()
emat 0.5.2, plotly 4.14.3
For this walkthrough of database features, we’ll work in a temporary directory. (In real projects you’ll likely want to save your data somewhere less ephemeral, so don’t just copy this tempfile code into your work.)
[2]:
import tempfile
tempdir = tempfile.TemporaryDirectory()
os.chdir(tempdir.name)
We begin our example by populating a database with some experimental data, by creating and running a single design of experiments for the Road Test model.
[3]:
import emat.examples
scope, db, model = emat.examples.road_test()
design = model.design_experiments()
model.run_experiments(design);
Single-Design Datasets¶
Writing Out Raw Data¶
When the database has only a single design of experiments, or if we don’t care about any differentiation between multiple designs that we may have created and ran, we can dump the entire set of model runs, including uncertainties, policy levers, and performance measures, all consolidated into a single pandas DataFrame using the read_experiment_all
function. The constants even appear in this DataFrame too, for good measure.
[4]:
df = db.read_experiment_all(scope.name)
df
[4]:
free_flow_time | initial_capacity | alpha | beta | input_flow | value_of_time | unit_cost_expansion | interest_rate | yield_curve | expand_capacity | amortization_period | debt_type | interest_rate_lock | no_build_travel_time | build_travel_time | time_savings | value_of_time_savings | net_benefits | cost_of_capacity_expansion | present_cost_expansion | |
---|---|---|---|---|---|---|---|---|---|---|---|---|---|---|---|---|---|---|---|---|
experiment | ||||||||||||||||||||
1 | 60 | 100 | 0.184682 | 5.237143 | 115 | 0.059510 | 118.213466 | 0.031645 | 0.015659 | 18.224793 | 38 | Rev Bond | False | 83.038716 | 69.586789 | 13.451927 | 92.059972 | -22.290905 | 114.350877 | 2154.415985 |
2 | 60 | 100 | 0.166133 | 4.121963 | 129 | 0.107772 | 141.322696 | 0.037612 | 0.007307 | 87.525790 | 36 | Paygo | True | 88.474313 | 62.132583 | 26.341730 | 366.219659 | -16.843014 | 383.062672 | 12369.380535 |
3 | 60 | 100 | 0.198937 | 4.719838 | 105 | 0.040879 | 97.783320 | 0.028445 | -0.001545 | 45.698048 | 44 | GO Bond | False | 75.027180 | 62.543328 | 12.483852 | 53.584943 | -113.988412 | 167.573355 | 4468.506839 |
4 | 60 | 100 | 0.158758 | 4.915816 | 113 | 0.182517 | 127.224901 | 0.036234 | 0.004342 | 51.297546 | 42 | GO Bond | True | 77.370428 | 62.268768 | 15.101660 | 311.462907 | 11.539561 | 299.923347 | 6526.325171 |
5 | 60 | 100 | 0.157671 | 3.845952 | 133 | 0.067102 | 107.820482 | 0.039257 | 0.001558 | 22.824149 | 42 | Paygo | False | 88.328990 | 72.848428 | 15.480561 | 138.156464 | 78.036616 | 60.119848 | 2460.910705 |
... | ... | ... | ... | ... | ... | ... | ... | ... | ... | ... | ... | ... | ... | ... | ... | ... | ... | ... | ... | ... |
106 | 60 | 100 | 0.169674 | 4.939898 | 150 | 0.131775 | 112.348054 | 0.033034 | -0.000120 | 24.215074 | 34 | Rev Bond | True | 135.446332 | 85.847986 | 49.598345 | 980.371960 | 841.462782 | 138.909178 | 2720.516457 |
107 | 60 | 100 | 0.148297 | 3.824779 | 110 | 0.103255 | 105.248708 | 0.033437 | 0.007041 | 38.013885 | 22 | GO Bond | True | 72.811506 | 63.736168 | 9.075338 | 103.078214 | -146.712793 | 249.791007 | 4000.912327 |
108 | 60 | 100 | 0.134701 | 3.627795 | 144 | 0.035233 | 132.063099 | 0.036702 | 0.018681 | 52.155613 | 32 | Paygo | True | 90.340993 | 66.617994 | 23.722999 | 120.358653 | -112.568104 | 232.926757 | 6887.831931 |
109 | 60 | 100 | 0.176125 | 4.243675 | 100 | 0.188515 | 97.503468 | 0.035896 | 0.016807 | 38.352260 | 33 | Paygo | True | 70.567477 | 62.664842 | 7.902635 | 148.976184 | 25.480553 | 123.495631 | 3739.478399 |
110 | 60 | 100 | 0.142843 | 4.486119 | 145 | 0.064909 | 99.837764 | 0.035372 | 0.011055 | 15.851006 | 46 | GO Bond | False | 105.386378 | 83.456509 | 21.929869 | 206.401068 | 127.311542 | 79.089526 | 1582.528991 |
110 rows × 20 columns
Exporting this data is simply a matter of using the usual pandas methods to save the dataframe to a format of your choosing. We’ll save our data into a gzipped CSV file, which is somewhat compressed (we’re not monsters here) but still widely compatible for a variety of uses.
[5]:
df.to_csv("road_test_1.csv.gz")
This table contains most of the information we want to export from our database, but not everything. We also probably want to have access to all of the information in the exploratory scope as well. Our example generator gives us a Scope
reference directly, but if we didn’t have that we can still extract it from the database, using the read_scope
method.
[6]:
s = db.read_scope()
s
[6]:
<emat.Scope with 2 constants, 7 uncertainties, 4 levers, 7 measures>
[7]:
s.dump(filename="road_test_scope.yaml")
[8]:
show_dir('.')
/
├── road_test_1.csv.gz
└── road_test_scope.yaml
Reading In Raw Data¶
Now, we’re ready to begin anew, constructing a fresh database from scratch, using only the raw formatted files.
First, let’s load our scope from the yaml file, and initialize a clean database using that scope.
[9]:
s2 = emat.Scope("road_test_scope.yaml")
[10]:
db2 = emat.SQLiteDB("road_test_2.sqldb")
[11]:
db2.store_scope(s2)
Just as we used pandas to save out our consolidated DataFrame of experimental results, we can use it to read in a consolidated table of experiments.
[12]:
df2 = pd.read_csv("road_test_1.csv.gz", index_col='experiment')
df2
[12]:
free_flow_time | initial_capacity | alpha | beta | input_flow | value_of_time | unit_cost_expansion | interest_rate | yield_curve | expand_capacity | amortization_period | debt_type | interest_rate_lock | no_build_travel_time | build_travel_time | time_savings | value_of_time_savings | net_benefits | cost_of_capacity_expansion | present_cost_expansion | |
---|---|---|---|---|---|---|---|---|---|---|---|---|---|---|---|---|---|---|---|---|
experiment | ||||||||||||||||||||
1 | 60 | 100 | 0.184682 | 5.237143 | 115 | 0.059510 | 118.213466 | 0.031645 | 0.015659 | 18.224793 | 38 | Rev Bond | False | 83.038716 | 69.586789 | 13.451927 | 92.059972 | -22.290905 | 114.350877 | 2154.415985 |
2 | 60 | 100 | 0.166133 | 4.121963 | 129 | 0.107772 | 141.322696 | 0.037612 | 0.007307 | 87.525790 | 36 | Paygo | True | 88.474313 | 62.132583 | 26.341730 | 366.219659 | -16.843014 | 383.062672 | 12369.380535 |
3 | 60 | 100 | 0.198937 | 4.719838 | 105 | 0.040879 | 97.783320 | 0.028445 | -0.001545 | 45.698048 | 44 | GO Bond | False | 75.027180 | 62.543328 | 12.483852 | 53.584943 | -113.988412 | 167.573355 | 4468.506839 |
4 | 60 | 100 | 0.158758 | 4.915816 | 113 | 0.182517 | 127.224901 | 0.036234 | 0.004342 | 51.297546 | 42 | GO Bond | True | 77.370428 | 62.268768 | 15.101660 | 311.462907 | 11.539561 | 299.923347 | 6526.325171 |
5 | 60 | 100 | 0.157671 | 3.845952 | 133 | 0.067102 | 107.820482 | 0.039257 | 0.001558 | 22.824149 | 42 | Paygo | False | 88.328990 | 72.848428 | 15.480561 | 138.156464 | 78.036616 | 60.119848 | 2460.910705 |
... | ... | ... | ... | ... | ... | ... | ... | ... | ... | ... | ... | ... | ... | ... | ... | ... | ... | ... | ... | ... |
106 | 60 | 100 | 0.169674 | 4.939898 | 150 | 0.131775 | 112.348054 | 0.033034 | -0.000120 | 24.215074 | 34 | Rev Bond | True | 135.446332 | 85.847986 | 49.598345 | 980.371960 | 841.462782 | 138.909178 | 2720.516457 |
107 | 60 | 100 | 0.148297 | 3.824779 | 110 | 0.103255 | 105.248708 | 0.033437 | 0.007041 | 38.013885 | 22 | GO Bond | True | 72.811506 | 63.736168 | 9.075338 | 103.078214 | -146.712793 | 249.791007 | 4000.912327 |
108 | 60 | 100 | 0.134701 | 3.627795 | 144 | 0.035233 | 132.063099 | 0.036702 | 0.018681 | 52.155613 | 32 | Paygo | True | 90.340993 | 66.617994 | 23.722999 | 120.358653 | -112.568104 | 232.926757 | 6887.831931 |
109 | 60 | 100 | 0.176125 | 4.243675 | 100 | 0.188515 | 97.503468 | 0.035896 | 0.016807 | 38.352260 | 33 | Paygo | True | 70.567477 | 62.664842 | 7.902635 | 148.976184 | 25.480553 | 123.495631 | 3739.478399 |
110 | 60 | 100 | 0.142843 | 4.486119 | 145 | 0.064909 | 99.837764 | 0.035372 | 0.011055 | 15.851006 | 46 | GO Bond | False | 105.386378 | 83.456509 | 21.929869 | 206.401068 | 127.311542 | 79.089526 | 1582.528991 |
110 rows × 20 columns
Writing experiments to a database is not quite as simple as reading them. There is a parallel write_experiment_all
method for the Database
class, but to use it we need to provide not only the DataFrame of actual results, but also a name for the design of experiments we are writing (all experiments exist within designs) and the source of the performance measure results (zero means actual results from a core model run, and non-zero values are ID numbers for metamodels). This allows many
different possible sets of performance measures to be stored for the same set of input parameters.
[13]:
db2.write_experiment_all(
scope_name=s2.name,
design_name='general',
source=0,
xlm_df=df2,
)
[14]:
display_experiments(s2, 'general', db=db2, rows=['time_savings'])
Time Savings
Multiple-Design Datasets¶
The EMAT database is not limited to storing a single design of experiments. Multiple designs can be stored for the same scope. We’ll add a set of univariate sensitivity test to our database, and a “ref” design that contains a single experiment with all inputs set to their default values.
[15]:
design_uni = model.design_experiments(sampler='uni')
model.run_experiments(design_uni)
model.run_reference_experiment();
We now have three designs stored in our database. We can confirm this by reading out the set of design names.
[16]:
db.read_design_names(s.name)
[16]:
['lhs', 'ref', 'uni']
The design names we se here are the default names given when designs are created with each of the given samplers. When creating new designs, we can override the default names with other names of our choice using the design_name
argument. The names can be any string not already in use.
[17]:
design_b = model.design_experiments(sampler='lhs', design_name='bruce')
db.read_design_names(s.name)
[17]:
['bruce', 'lhs', 'ref', 'uni']
If you try to re-use a name you’ll get an error, as having multiple designs with the same name does not allow you to make it clear which design you are referring to.
[18]:
try:
model.design_experiments(sampler='lhs', design_name='bruce')
except ValueError as err:
print(err)
the design "bruce" already exists for scope "EMAT Road Test"
As noted above, the design name, which can be any string, is separate from the sampler method. A default design name based on the name of the sampler method is used if no design name is given. The selected sampler must be one available in EMAT, as the sampler defines a particular logic about how to generate the design.
[19]:
try:
model.design_experiments(sampler='uni')
except ValueError as err:
print(err)
Note that there can be some experiments that are in more than one design. This is not merely duplicating the experiment and results, but actually assigning the same experiment to both designs. We can see this for the ‘uni’ and ‘ref’ designs – both contain the all-default parameters experiment, and when we read these designs out of the database, the same experiment number is reported out in both designs.
[20]:
db.read_experiment_all(scope.name, design_name='uni').head()
[20]:
free_flow_time | initial_capacity | alpha | beta | input_flow | value_of_time | unit_cost_expansion | interest_rate | yield_curve | expand_capacity | amortization_period | debt_type | interest_rate_lock | no_build_travel_time | build_travel_time | time_savings | value_of_time_savings | net_benefits | cost_of_capacity_expansion | present_cost_expansion | |
---|---|---|---|---|---|---|---|---|---|---|---|---|---|---|---|---|---|---|---|---|
experiment | ||||||||||||||||||||
111 | 60 | 100 | 0.15 | 4.0 | 100 | 0.075 | 100.0 | 0.03 | 0.01 | 10.0 | 30 | GO Bond | False | 69.0 | 66.147121 | 2.852879 | 21.396592 | -30.807346 | 52.203937 | 1000.0 |
112 | 60 | 100 | 0.10 | 4.0 | 100 | 0.075 | 100.0 | 0.03 | 0.01 | 10.0 | 30 | GO Bond | False | 66.0 | 64.098081 | 1.901919 | 14.264395 | -37.939543 | 52.203937 | 1000.0 |
113 | 60 | 100 | 0.20 | 4.0 | 100 | 0.075 | 100.0 | 0.03 | 0.01 | 10.0 | 30 | GO Bond | False | 72.0 | 68.196161 | 3.803839 | 28.528789 | -23.675148 | 52.203937 | 1000.0 |
114 | 60 | 100 | 0.15 | 3.5 | 100 | 0.075 | 100.0 | 0.03 | 0.01 | 10.0 | 30 | GO Bond | False | 69.0 | 66.447155 | 2.552845 | 19.146338 | -33.057600 | 52.203937 | 1000.0 |
115 | 60 | 100 | 0.15 | 5.5 | 100 | 0.075 | 100.0 | 0.03 | 0.01 | 10.0 | 30 | GO Bond | False | 69.0 | 65.328227 | 3.671773 | 27.538295 | -24.665642 | 52.203937 | 1000.0 |
[21]:
db.read_experiment_all(scope.name, design_name='ref')
[21]:
free_flow_time | initial_capacity | alpha | beta | input_flow | value_of_time | unit_cost_expansion | interest_rate | yield_curve | expand_capacity | amortization_period | debt_type | interest_rate_lock | no_build_travel_time | build_travel_time | time_savings | value_of_time_savings | net_benefits | cost_of_capacity_expansion | present_cost_expansion | |
---|---|---|---|---|---|---|---|---|---|---|---|---|---|---|---|---|---|---|---|---|
experiment | ||||||||||||||||||||
111 | 60 | 100 | 0.15 | 4.0 | 100 | 0.075 | 100.0 | 0.03 | 0.01 | 10.0 | 30 | GO Bond | False | 69.0 | 66.147121 | 2.852879 | 21.396592 | -30.807346 | 52.203937 | 1000.0 |
One “gotcha” to be wary of is unintentionally replicating experiments. By default, the random_seed
for randomly generated experiemnts is set to 0 for reproducibility. This means that, for example, the ‘bruce’ design is actually the same as the original ‘lhs’ design:
[22]:
db.read_experiment_all(scope.name, design_name='lhs').equals(
db.read_experiment_all(scope.name, design_name='bruce')
)
[22]:
True
If we want a new set of random experiments with the same sampler and other parameters, we’ll need to provide a different random_seed
.
[23]:
design_b = model.design_experiments(sampler='lhs', design_name='new_bruce', random_seed=42)
db.read_experiment_all(scope.name, design_name='lhs').equals(design_b)
[23]:
False
Writing Out Raw Data¶
We can read a single dataframe containing all the experiments associated with this scope by omitting the design_name
argument, just as if there was only one design.
[24]:
df = db.read_experiment_all(scope.name)
df
[24]:
free_flow_time | initial_capacity | alpha | beta | input_flow | value_of_time | unit_cost_expansion | interest_rate | yield_curve | expand_capacity | amortization_period | debt_type | interest_rate_lock | no_build_travel_time | build_travel_time | time_savings | value_of_time_savings | net_benefits | cost_of_capacity_expansion | present_cost_expansion | |
---|---|---|---|---|---|---|---|---|---|---|---|---|---|---|---|---|---|---|---|---|
experiment | ||||||||||||||||||||
1 | 60 | 100 | 0.184682 | 5.237143 | 115 | 0.059510 | 118.213466 | 0.031645 | 0.015659 | 18.224793 | 38 | Rev Bond | False | 83.038716 | 69.586789 | 13.451927 | 92.059972 | -22.290905 | 114.350877 | 2154.415985 |
2 | 60 | 100 | 0.166133 | 4.121963 | 129 | 0.107772 | 141.322696 | 0.037612 | 0.007307 | 87.525790 | 36 | Paygo | True | 88.474313 | 62.132583 | 26.341730 | 366.219659 | -16.843014 | 383.062672 | 12369.380535 |
3 | 60 | 100 | 0.198937 | 4.719838 | 105 | 0.040879 | 97.783320 | 0.028445 | -0.001545 | 45.698048 | 44 | GO Bond | False | 75.027180 | 62.543328 | 12.483852 | 53.584943 | -113.988412 | 167.573355 | 4468.506839 |
4 | 60 | 100 | 0.158758 | 4.915816 | 113 | 0.182517 | 127.224901 | 0.036234 | 0.004342 | 51.297546 | 42 | GO Bond | True | 77.370428 | 62.268768 | 15.101660 | 311.462907 | 11.539561 | 299.923347 | 6526.325171 |
5 | 60 | 100 | 0.157671 | 3.845952 | 133 | 0.067102 | 107.820482 | 0.039257 | 0.001558 | 22.824149 | 42 | Paygo | False | 88.328990 | 72.848428 | 15.480561 | 138.156464 | 78.036616 | 60.119848 | 2460.910705 |
... | ... | ... | ... | ... | ... | ... | ... | ... | ... | ... | ... | ... | ... | ... | ... | ... | ... | ... | ... | ... |
238 | 60 | 100 | 0.164649 | 4.851786 | 104 | 0.012456 | 127.667298 | 0.025673 | 0.012674 | 24.114264 | 30 | GO Bond | True | NaN | NaN | NaN | NaN | NaN | NaN | NaN |
239 | 60 | 100 | 0.113568 | 4.545709 | 105 | 0.137105 | 139.911784 | 0.028280 | 0.016936 | 92.883345 | 15 | GO Bond | True | NaN | NaN | NaN | NaN | NaN | NaN | NaN |
240 | 60 | 100 | 0.184434 | 4.732430 | 144 | 0.100242 | 99.904559 | 0.030392 | 0.019534 | 84.793007 | 15 | GO Bond | True | NaN | NaN | NaN | NaN | NaN | NaN | NaN |
241 | 60 | 100 | 0.146598 | 3.616301 | 107 | 0.065130 | 135.593036 | 0.035733 | 0.002085 | 29.107628 | 34 | Paygo | True | NaN | NaN | NaN | NaN | NaN | NaN | NaN |
242 | 60 | 100 | 0.193327 | 5.402220 | 134 | 0.048285 | 140.945872 | 0.026210 | -0.001255 | 26.285726 | 44 | Rev Bond | False | NaN | NaN | NaN | NaN | NaN | NaN | NaN |
242 rows × 20 columns
This dataframe is different than the one we saw earlier with the same command, as we have since added a few more experiments to the database in a few different designs. If we don’t give a design_name
argument, we’ll retrieve every (unique) experiment from every design currently stored in the database.
[25]:
df.to_csv("road_test_2.csv.gz")
If we want to be able to reconstruct the various designs of experiments later, we’ll also need to write out instructions for that. The read_all_experiment_ids
method can give us a dictionary of all the relevant information.
[26]:
design_experiments = db.read_all_experiment_ids(scope.name, design_name='*',grouped=True)
design_experiments
[26]:
{'bruce': '1-110',
'lhs': '1-110',
'new_bruce': '133-242',
'ref': '111',
'uni': '111-132',
'uni_2': '111-132'}
We can write this dictionary to a file in ‘yaml’ format.
[27]:
with open("road_test_design_experiments.yaml", 'wt') as f:
yaml.dump(design_experiments, f)
Reading In Raw Data¶
To construct a new emat Database with multiple designs of experients,…
[28]:
db3 = emat.SQLiteDB("road_test_3.sqldb")
db3.store_scope(s2)
[29]:
df3 = pd.read_csv("road_test_2.csv.gz", index_col='experiment')
df3
[29]:
free_flow_time | initial_capacity | alpha | beta | input_flow | value_of_time | unit_cost_expansion | interest_rate | yield_curve | expand_capacity | amortization_period | debt_type | interest_rate_lock | no_build_travel_time | build_travel_time | time_savings | value_of_time_savings | net_benefits | cost_of_capacity_expansion | present_cost_expansion | |
---|---|---|---|---|---|---|---|---|---|---|---|---|---|---|---|---|---|---|---|---|
experiment | ||||||||||||||||||||
1 | 60 | 100 | 0.184682 | 5.237143 | 115 | 0.059510 | 118.213466 | 0.031645 | 0.015659 | 18.224793 | 38 | Rev Bond | False | 83.038716 | 69.586789 | 13.451927 | 92.059972 | -22.290905 | 114.350877 | 2154.415985 |
2 | 60 | 100 | 0.166133 | 4.121963 | 129 | 0.107772 | 141.322696 | 0.037612 | 0.007307 | 87.525790 | 36 | Paygo | True | 88.474313 | 62.132583 | 26.341730 | 366.219659 | -16.843014 | 383.062672 | 12369.380535 |
3 | 60 | 100 | 0.198937 | 4.719838 | 105 | 0.040879 | 97.783320 | 0.028445 | -0.001545 | 45.698048 | 44 | GO Bond | False | 75.027180 | 62.543328 | 12.483852 | 53.584943 | -113.988412 | 167.573355 | 4468.506839 |
4 | 60 | 100 | 0.158758 | 4.915816 | 113 | 0.182517 | 127.224901 | 0.036234 | 0.004342 | 51.297546 | 42 | GO Bond | True | 77.370428 | 62.268768 | 15.101660 | 311.462907 | 11.539561 | 299.923347 | 6526.325171 |
5 | 60 | 100 | 0.157671 | 3.845952 | 133 | 0.067102 | 107.820482 | 0.039257 | 0.001558 | 22.824149 | 42 | Paygo | False | 88.328990 | 72.848428 | 15.480561 | 138.156464 | 78.036616 | 60.119848 | 2460.910705 |
... | ... | ... | ... | ... | ... | ... | ... | ... | ... | ... | ... | ... | ... | ... | ... | ... | ... | ... | ... | ... |
238 | 60 | 100 | 0.164649 | 4.851786 | 104 | 0.012456 | 127.667298 | 0.025673 | 0.012674 | 24.114264 | 30 | GO Bond | True | NaN | NaN | NaN | NaN | NaN | NaN | NaN |
239 | 60 | 100 | 0.113568 | 4.545709 | 105 | 0.137105 | 139.911784 | 0.028280 | 0.016936 | 92.883345 | 15 | GO Bond | True | NaN | NaN | NaN | NaN | NaN | NaN | NaN |
240 | 60 | 100 | 0.184434 | 4.732430 | 144 | 0.100242 | 99.904559 | 0.030392 | 0.019534 | 84.793007 | 15 | GO Bond | True | NaN | NaN | NaN | NaN | NaN | NaN | NaN |
241 | 60 | 100 | 0.146598 | 3.616301 | 107 | 0.065130 | 135.593036 | 0.035733 | 0.002085 | 29.107628 | 34 | Paygo | True | NaN | NaN | NaN | NaN | NaN | NaN | NaN |
242 | 60 | 100 | 0.193327 | 5.402220 | 134 | 0.048285 | 140.945872 | 0.026210 | -0.001255 | 26.285726 | 44 | Rev Bond | False | NaN | NaN | NaN | NaN | NaN | NaN | NaN |
242 rows × 20 columns
[30]:
with open("road_test_design_experiments.yaml", 'rt') as f:
design_experiments2 = yaml.safe_load(f)
design_experiments2
[30]:
{'bruce': '1-110',
'lhs': '1-110',
'new_bruce': '133-242',
'ref': '111',
'uni': '111-132',
'uni_2': '111-132'}
[31]:
db3.write_experiment_all(
scope_name=s2.name,
design_name=design_experiments2,
source=0,
xlm_df=df3,
)
[32]:
db3.read_design_names(s.name)
[32]:
['bruce', 'lhs', 'new_bruce', 'ref', 'uni', 'uni_2']
[33]:
db3.read_all_experiment_ids(scope.name, design_name='*',grouped=True)
[33]:
{'bruce': '1-110',
'lhs': '1-110',
'new_bruce': '133-242',
'ref': '111',
'uni': '111-132',
'uni_2': '111-132'}
[34]:
db3.read_experiment_all(scope.name, design_name='uni').head()
[34]:
free_flow_time | initial_capacity | alpha | beta | input_flow | value_of_time | unit_cost_expansion | interest_rate | yield_curve | expand_capacity | amortization_period | debt_type | interest_rate_lock | no_build_travel_time | build_travel_time | time_savings | value_of_time_savings | net_benefits | cost_of_capacity_expansion | present_cost_expansion | |
---|---|---|---|---|---|---|---|---|---|---|---|---|---|---|---|---|---|---|---|---|
experiment | ||||||||||||||||||||
111 | 60 | 100 | 0.15 | 4.0 | 100 | 0.075 | 100.0 | 0.03 | 0.01 | 10.0 | 30 | GO Bond | False | 69.0 | 66.147121 | 2.852879 | 21.396592 | -30.807346 | 52.203937 | 1000.0 |
112 | 60 | 100 | 0.10 | 4.0 | 100 | 0.075 | 100.0 | 0.03 | 0.01 | 10.0 | 30 | GO Bond | False | 66.0 | 64.098081 | 1.901919 | 14.264395 | -37.939543 | 52.203937 | 1000.0 |
113 | 60 | 100 | 0.20 | 4.0 | 100 | 0.075 | 100.0 | 0.03 | 0.01 | 10.0 | 30 | GO Bond | False | 72.0 | 68.196161 | 3.803839 | 28.528789 | -23.675148 | 52.203937 | 1000.0 |
114 | 60 | 100 | 0.15 | 3.5 | 100 | 0.075 | 100.0 | 0.03 | 0.01 | 10.0 | 30 | GO Bond | False | 69.0 | 66.447155 | 2.552845 | 19.146338 | -33.057600 | 52.203937 | 1000.0 |
115 | 60 | 100 | 0.15 | 5.5 | 100 | 0.075 | 100.0 | 0.03 | 0.01 | 10.0 | 30 | GO Bond | False | 69.0 | 65.328227 | 3.671773 | 27.538295 | -24.665642 | 52.203937 | 1000.0 |
Re-running Experiments¶
This section provides a short walkthrough of how to handle mistakes in an EMAT database. By “mistakes” we are referring to incorrect values that have been written into the database by accident, generally arising from core model runs that were misconfigured or suffered non-fatal errors that caused the results to be invalid.
One approach to handling such problems is to simply start over with a brand new clean database file. However, this may be inconvenient if the database already includes a number of valid results, especially if those valid results were expensive to generate. It may also be desirable to keep prior invalid results on hand, so as to easily recognized when errors recur.
We begin this example by populating our database with some more experimental data, by creating and running a single design of experiments for the Road Test model, except these experiments will be created with a misconfigured model (lane_width = 11, it should be 10), so the results will be bad. (In general, you probably won’t intentionally create corrupt data, but we’re doing so here for expository purposes, so we’ll give this design a name of ‘oops’ so we can readily recall what we’ve done.)
[35]:
model.lane_width = 10.3
oops = model.design_experiments(design_name='oops', random_seed=12345)
model.run_experiments(oops);
We can review a dataframe of results as before, using the read_experiment_all
method. This time we will add with_run_ids=True
, which will add an extra column to the index, showing a universally unique id attached to each row of results.
[36]:
oops_result1 = db.read_experiment_all(scope.name, 'oops', with_run_ids=True)
oops_result1.head()
[36]:
free_flow_time | initial_capacity | alpha | beta | input_flow | value_of_time | unit_cost_expansion | interest_rate | yield_curve | expand_capacity | amortization_period | debt_type | interest_rate_lock | no_build_travel_time | build_travel_time | time_savings | value_of_time_savings | net_benefits | cost_of_capacity_expansion | present_cost_expansion | ||
---|---|---|---|---|---|---|---|---|---|---|---|---|---|---|---|---|---|---|---|---|---|
experiment | run | ||||||||||||||||||||
243 | b2358b5e-be73-11eb-809d-acde48001122 | 60 | 100 | 0.157955 | 4.270404 | 112 | 0.151487 | 116.445987 | 0.029784 | 0.018968 | 50.163393 | 38 | Rev Bond | False | 75.376818 | 79.495869 | -4.119051 | -69.886026 | -381.303111 | 311.417085 | 5841.325841 |
244 | b2376db6-be73-11eb-809d-acde48001122 | 60 | 100 | 0.165144 | 4.008218 | 134 | 0.039670 | 102.879244 | 0.032505 | 0.000708 | 57.330143 | 40 | Rev Bond | False | 92.024075 | 83.059349 | 8.964726 | 47.654780 | -220.075486 | 267.730266 | 5898.081794 |
245 | b238ff32-be73-11eb-809d-acde48001122 | 60 | 100 | 0.103964 | 4.019636 | 92 | 0.100936 | 131.282035 | 0.038432 | 0.002658 | 64.949710 | 39 | GO Bond | True | 64.461446 | 77.449591 | -12.988145 | -120.608948 | -520.723349 | 400.114402 | 8526.730135 |
246 | b23a6b42-be73-11eb-809d-acde48001122 | 60 | 100 | 0.172162 | 4.920619 | 144 | 0.135484 | 95.348634 | 0.025803 | 0.008870 | 77.498534 | 26 | Paygo | False | 122.134147 | 80.716842 | 41.417305 | 808.041389 | 513.014691 | 295.026699 | 7389.379292 |
247 | b23bdc66-be73-11eb-809d-acde48001122 | 60 | 100 | 0.136954 | 4.901048 | 100 | 0.109919 | 114.310247 | 0.027538 | 0.016733 | 46.312847 | 41 | Paygo | False | 68.217253 | 78.289223 | -10.071970 | -110.710261 | -276.303370 | 165.593109 | 5294.033017 |
[37]:
display_experiments(scope, oops_result1, rows=['time_savings'])
Time Savings
Some of these results are obviously problematic. Increasing capacity cannot possibly result in a negative travel time savings. (Braess paradox doesn’t apply here because it’s just one link, not a network.) So those negative values are clearly wrong. We can fix the model so they won’t be wrong, but by default the run_experiments
method won’t actually re-run models when the results are already available in the database. To solve this conundrum, we can mark the incorrect results as invalid,
using a query to pull out the rows that can be flagged as wrong.
[38]:
db.invalidate_experiment_runs(
queries=['time_savings < 0']
)
[38]:
[73]
The [73]
returned here indicates that 73 sets of results were invalidated by this command. The invalidation command actually sets a “valid” flag in the database to False for these experiment runs, so that a persistant record that they are bad is stored in the database. Now we can fix our model, and then use the run_experiments
method to get new model runs for the invalidated results.
[39]:
model.lane_width = 10
oops_result2 = model.run_experiments(oops)
[40]:
display_experiments(scope, 'oops', db=db, rows=['time_savings'])
Time Savings
The re-run fixed the negative values, although it left in place the other experimental runs in the database. By the way we constructed this example, we know those are wrong too, and it’s evident in the apparent discontinuity in the input flow graph, which we can zoom in on.
[41]:
ax = oops_result2.plot.scatter(x='input_flow', y='time_savings', color='r')
ax.plot([109,135], [0,35], '--',color='y');
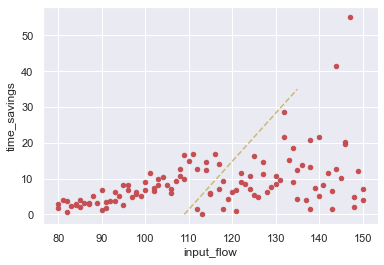
Those original results are bad too, and we want to invalidate them as well. In addition to giving conditional queries to the invalidate_experiment_runs
method, we can also give a dataframe of results that have run ids attached, and those unique ids will be used to to find and invalidate results in the database. Here, we pass in the dataframe of all the results, which contains all 110 runs, but only 37 runs are newly invalidated (77 were invalidated previously).
[42]:
db.invalidate_experiment_runs(
oops_result1
)
[42]:
37
Now when we run the experiments again, those 37 experiments are re-run.
[43]:
oops_result3 = model.run_experiments(oops)
[44]:
display_experiments(scope, 'lhs', db=db, rows=['time_savings'])
Time Savings
Writing Out All Runs¶
By default, the read_experiment_all
method returns the most recent valid set of performance measures for each experiment, but we can override this behavior to ask for 'all'
run results, or all 'valid'
or 'invalid'
results, by setting the runs
argument to those literal values. This allows us to easily write out data files containing all the results stored in the database.
[45]:
db.read_experiment_all(scope.name, with_run_ids=True, runs='all')
[45]:
free_flow_time | initial_capacity | alpha | beta | input_flow | value_of_time | unit_cost_expansion | interest_rate | yield_curve | expand_capacity | amortization_period | debt_type | interest_rate_lock | no_build_travel_time | build_travel_time | time_savings | value_of_time_savings | net_benefits | cost_of_capacity_expansion | present_cost_expansion | ||
---|---|---|---|---|---|---|---|---|---|---|---|---|---|---|---|---|---|---|---|---|---|
experiment | run | ||||||||||||||||||||
1 | af00ae82-be73-11eb-809d-acde48001122 | 60 | 100 | 0.184682 | 5.237143 | 115 | 0.059510 | 118.213466 | 0.031645 | 0.015659 | 18.224793 | 38 | Rev Bond | False | 83.038716 | 69.586789 | 13.451927 | 92.059972 | -22.290905 | 114.350877 | 2154.415985 |
2 | af0320e0-be73-11eb-809d-acde48001122 | 60 | 100 | 0.166133 | 4.121963 | 129 | 0.107772 | 141.322696 | 0.037612 | 0.007307 | 87.525790 | 36 | Paygo | True | 88.474313 | 62.132583 | 26.341730 | 366.219659 | -16.843014 | 383.062672 | 12369.380535 |
3 | af04aaaa-be73-11eb-809d-acde48001122 | 60 | 100 | 0.198937 | 4.719838 | 105 | 0.040879 | 97.783320 | 0.028445 | -0.001545 | 45.698048 | 44 | GO Bond | False | 75.027180 | 62.543328 | 12.483852 | 53.584943 | -113.988412 | 167.573355 | 4468.506839 |
4 | af0628e4-be73-11eb-809d-acde48001122 | 60 | 100 | 0.158758 | 4.915816 | 113 | 0.182517 | 127.224901 | 0.036234 | 0.004342 | 51.297546 | 42 | GO Bond | True | 77.370428 | 62.268768 | 15.101660 | 311.462907 | 11.539561 | 299.923347 | 6526.325171 |
5 | af07aea8-be73-11eb-809d-acde48001122 | 60 | 100 | 0.157671 | 3.845952 | 133 | 0.067102 | 107.820482 | 0.039257 | 0.001558 | 22.824149 | 42 | Paygo | False | 88.328990 | 72.848428 | 15.480561 | 138.156464 | 78.036616 | 60.119848 | 2460.910705 |
... | ... | ... | ... | ... | ... | ... | ... | ... | ... | ... | ... | ... | ... | ... | ... | ... | ... | ... | ... | ... | ... |
350 | b3f00848-be73-11eb-809d-acde48001122 | 60 | 100 | 0.127109 | 4.855843 | 103 | 0.130109 | 110.140882 | 0.039189 | 0.016098 | 69.050648 | 31 | GO Bond | False | 68.803652 | 60.687779 | 8.115873 | 108.762921 | -345.671302 | 454.434223 | 7605.299268 |
351 | b2d23300-be73-11eb-809d-acde48001122 | 60 | 100 | 0.191902 | 4.144514 | 102 | 0.145524 | 141.761624 | 0.037483 | 0.004611 | 20.006234 | 45 | GO Bond | True | 72.499003 | 83.787080 | -11.288077 | -167.553833 | -295.911196 | 128.357363 | 2836.116224 |
b3f17d7c-be73-11eb-809d-acde48001122 | 60 | 100 | 0.191902 | 4.144514 | 102 | 0.145524 | 141.761624 | 0.037483 | 0.004611 | 20.006234 | 45 | GO Bond | True | 72.499003 | 65.869676 | 6.629328 | 98.401992 | -29.955371 | 128.357363 | 2836.116224 | |
352 | b2d3b568-be73-11eb-809d-acde48001122 | 60 | 100 | 0.189252 | 4.588903 | 111 | 0.191116 | 105.423753 | 0.038912 | 0.012596 | 70.999408 | 16 | Rev Bond | True | 78.330479 | 78.364867 | -0.034388 | -0.729512 | -597.920964 | 597.191453 | 7485.024087 |
b3f2fdd2-be73-11eb-809d-acde48001122 | 60 | 100 | 0.189252 | 4.588903 | 111 | 0.191116 | 105.423753 | 0.038912 | 0.012596 | 70.999408 | 16 | Rev Bond | True | 78.330479 | 61.563090 | 16.767389 | 355.700934 | -241.490519 | 597.191453 | 7485.024087 |
462 rows × 20 columns
In the resulting dataframe above, we can see that we have retrieved two different runs for some of the experiments. Only one of them is valid for each. If we want to get all the stored runs and also mark the valid and invalid runs, we can read them seperately and attach a tag to the two dataframes.
[46]:
runs_1 = db.read_experiment_all(scope.name, with_run_ids=True, runs='valid')
runs_1['is_valid'] = True
runs_0 = db.read_experiment_all(scope.name, with_run_ids=True, runs='invalid', only_with_measures=True)
runs_0['is_valid'] = False
all_runs = pd.concat([runs_1, runs_0])
all_runs.sort_index()
[46]:
free_flow_time | initial_capacity | alpha | beta | input_flow | value_of_time | unit_cost_expansion | interest_rate | yield_curve | expand_capacity | ... | debt_type | interest_rate_lock | no_build_travel_time | build_travel_time | time_savings | value_of_time_savings | net_benefits | cost_of_capacity_expansion | present_cost_expansion | is_valid | ||
---|---|---|---|---|---|---|---|---|---|---|---|---|---|---|---|---|---|---|---|---|---|---|
experiment | run | |||||||||||||||||||||
1 | af00ae82-be73-11eb-809d-acde48001122 | 60 | 100 | 0.184682 | 5.237143 | 115 | 0.059510 | 118.213466 | 0.031645 | 0.015659 | 18.224793 | ... | Rev Bond | False | 83.038716 | 69.586789 | 13.451927 | 92.059972 | -22.290905 | 114.350877 | 2154.415985 | True |
2 | af0320e0-be73-11eb-809d-acde48001122 | 60 | 100 | 0.166133 | 4.121963 | 129 | 0.107772 | 141.322696 | 0.037612 | 0.007307 | 87.525790 | ... | Paygo | True | 88.474313 | 62.132583 | 26.341730 | 366.219659 | -16.843014 | 383.062672 | 12369.380535 | True |
3 | af04aaaa-be73-11eb-809d-acde48001122 | 60 | 100 | 0.198937 | 4.719838 | 105 | 0.040879 | 97.783320 | 0.028445 | -0.001545 | 45.698048 | ... | GO Bond | False | 75.027180 | 62.543328 | 12.483852 | 53.584943 | -113.988412 | 167.573355 | 4468.506839 | True |
4 | af0628e4-be73-11eb-809d-acde48001122 | 60 | 100 | 0.158758 | 4.915816 | 113 | 0.182517 | 127.224901 | 0.036234 | 0.004342 | 51.297546 | ... | GO Bond | True | 77.370428 | 62.268768 | 15.101660 | 311.462907 | 11.539561 | 299.923347 | 6526.325171 | True |
5 | af07aea8-be73-11eb-809d-acde48001122 | 60 | 100 | 0.157671 | 3.845952 | 133 | 0.067102 | 107.820482 | 0.039257 | 0.001558 | 22.824149 | ... | Paygo | False | 88.328990 | 72.848428 | 15.480561 | 138.156464 | 78.036616 | 60.119848 | 2460.910705 | True |
... | ... | ... | ... | ... | ... | ... | ... | ... | ... | ... | ... | ... | ... | ... | ... | ... | ... | ... | ... | ... | ... | ... |
350 | b3f00848-be73-11eb-809d-acde48001122 | 60 | 100 | 0.127109 | 4.855843 | 103 | 0.130109 | 110.140882 | 0.039189 | 0.016098 | 69.050648 | ... | GO Bond | False | 68.803652 | 60.687779 | 8.115873 | 108.762921 | -345.671302 | 454.434223 | 7605.299268 | True |
351 | b2d23300-be73-11eb-809d-acde48001122 | 60 | 100 | 0.191902 | 4.144514 | 102 | 0.145524 | 141.761624 | 0.037483 | 0.004611 | 20.006234 | ... | GO Bond | True | 72.499003 | 83.787080 | -11.288077 | -167.553833 | -295.911196 | 128.357363 | 2836.116224 | False |
b3f17d7c-be73-11eb-809d-acde48001122 | 60 | 100 | 0.191902 | 4.144514 | 102 | 0.145524 | 141.761624 | 0.037483 | 0.004611 | 20.006234 | ... | GO Bond | True | 72.499003 | 65.869676 | 6.629328 | 98.401992 | -29.955371 | 128.357363 | 2836.116224 | True | |
352 | b2d3b568-be73-11eb-809d-acde48001122 | 60 | 100 | 0.189252 | 4.588903 | 111 | 0.191116 | 105.423753 | 0.038912 | 0.012596 | 70.999408 | ... | Rev Bond | True | 78.330479 | 78.364867 | -0.034388 | -0.729512 | -597.920964 | 597.191453 | 7485.024087 | False |
b3f2fdd2-be73-11eb-809d-acde48001122 | 60 | 100 | 0.189252 | 4.588903 | 111 | 0.191116 | 105.423753 | 0.038912 | 0.012596 | 70.999408 | ... | Rev Bond | True | 78.330479 | 61.563090 | 16.767389 | 355.700934 | -241.490519 | 597.191453 | 7485.024087 | True |
462 rows × 21 columns
These mechanisms can be use to write out results of multiple runs, and to repopulate a database with both valid and invalid raw results. This can be done multiple ways (seperate files, one combined file, keeping track of invalidation queries, etc.). The particular implementations of each are left as an exercise for the reader.